It’s great when people talk about your brand – but not if they’re just saying how much they hate it. Most experienced marketers recognize by now that success is not measured by the number of people talking about your brand but the tone of those conversations. Sentiment analysis is one way of gauging the tone of that discourse.
Sentiment analysis is essentially a technologically-enabled way of measuring the tone of conversations about your brand. It’s sometimes referred to as ‘opinion mining’ and (in data science terms at least) it falls under the remit of natural language processing.
Sentiment analysis tools will scrape the web and social media networks to identify any chatter about your brand and then identify and extract any opinions being expressed.
It’s used for a variety of purposes: gauging the political mood, assessing the success of rebrands or new product launches, understanding how marketing campaigns are performing and seeing how well customer service is going across multiple channels and markets. All these applications share a common goal of using sentiment analysis to make more informed decisions based on public opinion.
Scalable feedback
In days when business was simpler, it was easier for a small business owner to understand their customer relations because they conducted or oversaw many of the transactions themselves. It was easier for local businesses to measure opinions because they rubbed shoulders with many of their customers.
Sentiment analysis is a useful tool for organisations too huge to easily measure everything that’s going on, in a world where conversations are so widely spread across complex channels that it’s impossible to oversee everything.
Sentiment analysis increases in complexity when multinational organisations want to get the same level of insights as companies that operate in just one language market. There’s been a great deal of activity to improve sentiment analysis in English-speaking markets and the available tools are now impressively accurate.
Add in the complexities of multiple languages and not as much work has yet been done. That’s particularly true for brands working in less common languages.
RELATED: Social Media Monitoring in International Markets |
For brands working in ‘mainstream’ languages – such as Spanish or French – there are generally either dedicated sentiment analysis tools for that language or reliable machine translation programs to use in combination with sentiment analysis programs.
If you’re trying to analyse brand opinion in languages that haven’t received as much attention, you may find the resources aren’t as available or reliable.
There’s some evidence that a combination of machine translation and sentiment analysis tools can be pretty effective. A 2014 study found that for Latvian – not a language with a huge amount of international resource – this approach worked well in lab conditions. The conclusion was that the quality of translation software was perfectly adequate to the task of analyzing sentiment in a body of tweet-type materials.
Perhaps the advantage of using machine translation in a project like this is that it doesn’t need to have a 100% accuracy rate. Machine translation isn’t always reliable but when applied to a large body of material it’s often accurate enough for the purposes of analyzing the flavor of opinion expressed across the body of content.
Furthermore, machine translation output is related to the availability of training data in a given language combination. Therefore, an English into Spanish engine is much more likely to produce better results than an English to Latvian engine, simply because there is more bilingual data in English to Spanish that can be used to train the machine translation engines.
If you’re trying to conduct sentiment analysis in multiple languages, particularly if they are less widely spoken languages, it’s advisable to include human translators in your project. This helps to check the validity of the data you’re using. A translator might be better able to pick up things like sarcasm, which a machine translation tool might easily misunderstand.
A new generation of sentiment analysis
Sentiment analysis has progressed to a point where it’s possible to ask relatively sophisticated questions to sentiment analysis programs. Opinion mining was formerly confined to the simple question of whether consumers generally think a brand is good or bad.
These days, it’s possible to delve a little deeper, looking into things such as general consumer intent or ask more granular questions about a specific product feature. It’s also possible to get real-time sentiment analysis, which should help you identify an emerging crisis or opportunity and respond quickly and effectively.
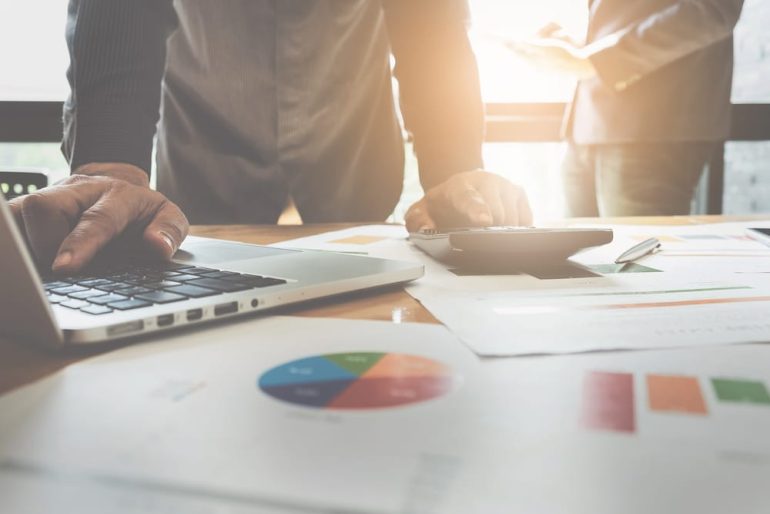
Data from sentiment analysis tools have the ability to influence your brand’s marketing or PR strategy and provide context to product feedback and customer service.
It goes without saying that time is of the essence when responding to negative criticisms about your brand. Failure to respond at the right time could be detrimental to future endeavors in the particular market you’re building a reputation in.
The whole point of sentiment analysis is that it’s scalable. In combination with natural language analysis, large-volume data analysis offers sweeping insights into the audience mindset and gives organisations the power to make better decisions as a result. But it’s by no means a given that organisations are effective at responding to the results of sentiment analysis projects.
Sentiment analysis is a worthless undertaking unless it results in real-world, actionable insights. There’s no point gauging the tone of customer opinion about your support function if you don’t intend to implement the required changes.
There’s no point monitoring sentiment in real-time if you don’t intend to rapidly adapt your PR to react. Before you spend money on sentiment analysis tools, make sure you have the resources in place to respond to what you find and that your organisation has the mindset to change in response to understanding consumer mood.