It wouldn’t be an exaggeration to say that machine translation has revolutionised the translation industry the way translation memory revolutionised it in the 90’s. Google, eBay, Microsoft and Amazon are all in a constant race trying to outdo each other in their MT efforts. With the machine translation landscape forever changing, is it possible to predict what lies ahead?
MT technology has gone through several generations, from rule-based and example-based MT, through statistical machine translation (SMT) to the latest neural machine translation (NMT). NMT has broken some of the barriers that previous generations of MT couldn’t overcome and therefore has created opportunities that weren’t there before.
Next generation of MT
The history of this technology would suggest that we are very likely to see yet another more innovative generation of MT in the future.
However, at this point in time, there is no grounded talk of any viable successor to NMT.
Whatever the next advances turn out to be, they are likely to come from companies like Amazon, Alibaba, Baidu, Facebook, Google or Microsoft. Especially in recent years, these players have been investing not only in offering more languages and increased quality but also in being able to produce translations faster.
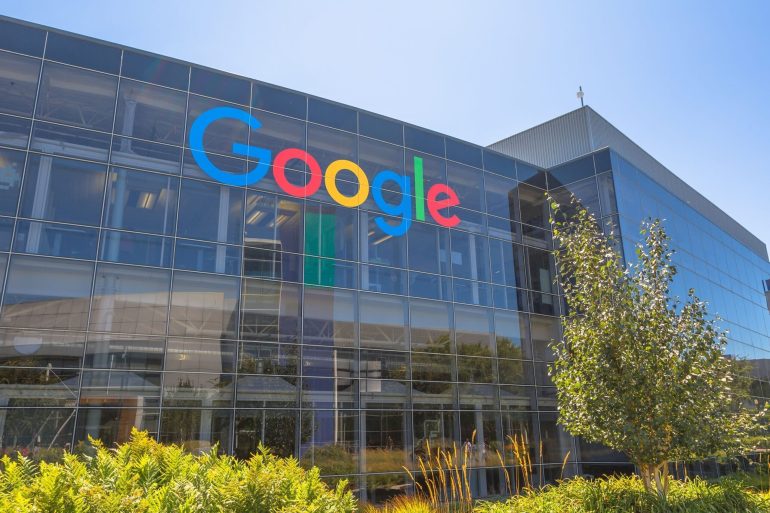
Google is known for its strong involvement in artifitical intelligence, which is not limited to machine translation. Editorial credit: Shutterstock.com
Google and Amazon have been working on incorporating translation and interpretation features into their smart assistants – Google Home and Alexa. Their next step is likely to focus on adapting the language output from the devices more to individual users in terms of the choice of words, the complexity of the language used and correctly understanding the meaning behind any ambiguous words spoken by the user.
Document-level attention
One of the major shortcomings of currently available MT systems is that they are not able to look beyond the sentence level. They translate sentences in isolation and do not go as far as being able to look at a document as a whole to work out context.
This means that machine translation can contain terminology inconsistencies, mix formal and informal ways of addressing the reader, as well as struggle with correctly interpreting references that link sentences two or more sentences together. That can include omitted pronouns that confuse the MT system.
Overcoming this would be a big step forward for a number of reasons.
Fewer inconsistencies and fewer mistranslations related to omitted pronouns would reduce the post-editing effort needed to polish the translation, and as a result, could allow translations to be published faster.
Automatic post-editing
Another field being explored as part of the efforts to reduce the need for post-editing, and which is likely to gain more attention in the near future, is Automatic Post-Editing or APE. In the APE process, there are two machines at work – one produces the translation and the second one performs the post-editing actions on that translation.
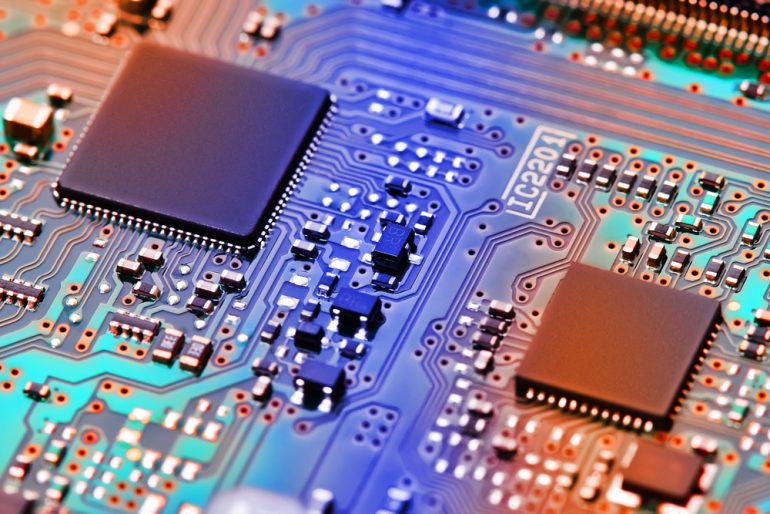
Automatic post-editing combines the power of two machines performing two different tasks.
This is possible thanks to the fact that the two machines are trained differently. The first one is trained in a standard way using previous translations. It learns the patterns governing both source and target languages and the correspondence between the two, which then allows it to produce best-guess translations for a given input sentence.
The second machine’s role is to post-edit the output generated by the first machine. It can be trained on the corrections made by humans to texts. In a similar way to the process described above, this machine learns any frequent patterns, which then makes it possible to apply those corrections to the MT output.
Even though APE has been around for a while, it’s safe to say its full potential still hasn’t been reached. The coming years might therefore bring some innovation to this field of machine learning.
Quality estimation
Another area ripe for exploration is machine translation quality estimation (MTQE). The QE step can be applied at the beginning of a translation workflow to predict the quality of the machine translation output for a given piece of text. This can happen before the machine translation reaches a human for post-editing, to give an idea of the effort that’s going to be required.
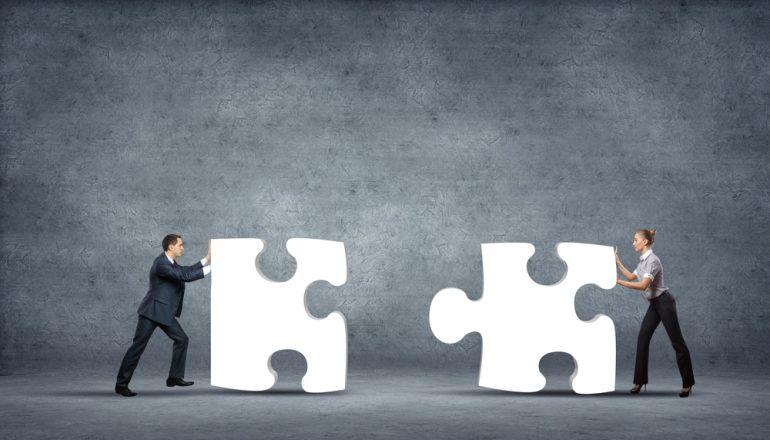
Unbabel, a company heavily relying on AI in providing its services, claims that “quality estimation is everything that is missing in machine translation”.
This quality estimation step would allow people to make more informed decisions, for instance whether the translation can be published immediately or whether it needs to be sent to a post-editor.
Although some CAT tools, Memsource for instance, and language services providers like Unbabel have been quick to embrace and incorporate MTQE features, there are still big questions regarding the reliability and consistency of the quality scores.
At times, this uncertainty prevents MTQE from wider adoption. Solving quality estimation could unlock further potential in MT, so we are bound to see a lot more research and resources being invested in making it a robust part of any localisation workflow that involves MT.
We can only wonder what the future of machine translation might bring. Whatever it turns out to be, it’s bound to continue making significant waves in the language industry.