In summary: How to implement machine translation into your translation process
- Language pairs – typically, languages that are geographically close will machine translate into each other more easily
- Custom data – if you already have a large number of good-quality translations, you can use that data to load into the machine translation engine and customise the output
- Quality management – you also need linguists who are trained in post-editing and a specialist team to manage quality using metrics that align with your objectives
It wasn’t long ago that most people thought machine translation was a cheaper, poor-quality version of the human-based translation process. Although statistical machine translation made great strides in quality compared to its rule-based predecessor, the launch of both Microsoft and Google’s Neural Machine Translation processes pushed machine translation from a niche add-on to a sought-after service.
Looking past the initial hype, neural machine translation (NMT) output proved to have some use in real-world scenarios. While it may not be 100% accurate and requires a particular level of checking and editing, it’s still good enough to speed up the translation process of certain types of content for a particular group of language pairs. Furthermore, based on the neural networks at its core, NMT is continually learning and constantly improving.
The sooner you use neural machine translation and the more you use it, the sooner you’ll start to see the benefits.
At TOPPAN Digital Language, we implemented neural machine translation in place of statistical machine translation in a variety of ways back in January 2018 and rapidly saw a jump in quality while also discovering more opportunities. This is what we learned during that process.
Things to consider
Implementing NMT is a journey. As a result, where you start is a huge part of the picture and largely dictates what you can start with. A common challenge is establishing a new process that will eventually scale up, and where you might be limited technically by what your existing content and translation systems can do.
First, you need to assess the overall quality to see how feasible it is to post-edit it to a ‘production-ready’ quality. Typically, there are two key factors that will give you a guide on whether machine translation is going to work on your content.
Language pairs – typically, languages that are geographically close will machine translate into each other more easily. This is due to the huge amounts of good quality data that is readily available and that all machine translation engines are trained with.
Essentially, machine translation between English, French, Spanish, Italian, German, Portuguese and Dutch will perform better compared to translation between English and Arabic, Chinese, Japanese, Korean or Russian – even before custom data is added.
Custom data – If you already have a large number of good-quality translations, you can use that data to load into the machine translation engine and customise the output. Any amount of data is worth including and the quality of improvement from custom data is one of the key areas of performance of neural machine translation. It’s also worth investing in getting your data into TMX format to help load it into the NMT engines.
If you are not convinced in the initial stage, you can always start with a small amount of data. However, you’ll need to make sure you load at least 20,000 segments of custom data from your past translations to start to see a difference.
Once you have your data ready, you can then run the customisation. Some tools can do this instantly, on the fly, while others need many hours to run the full customisation of the underlying model. Once the customisation is ready, you can then feed in some samples and have a linguist assess the output.
But before you start permanently incorporating linguists in the machine translation process, you need to tackle one of the biggest challenges in this process: communication with your linguists.
Managing expectations
With automation not showing any signs of slowing down in a wide range of industry sectors, translators have naturally been somewhat hostile to the notion of more automation in their work.
But it’s important to realise that with these advancements in machine translation, post-editing is slowly becoming a large part of the NMT process – which means more content to be translated by linguists. As a result, this actually increases the opportunities for linguists to diversify their skill sets.
If you want to persuade existing translators to incorporate post-editing, it’s worth noting that translating and post-editing content are two different skill sets – your translators will definitely require training to become post-editors.
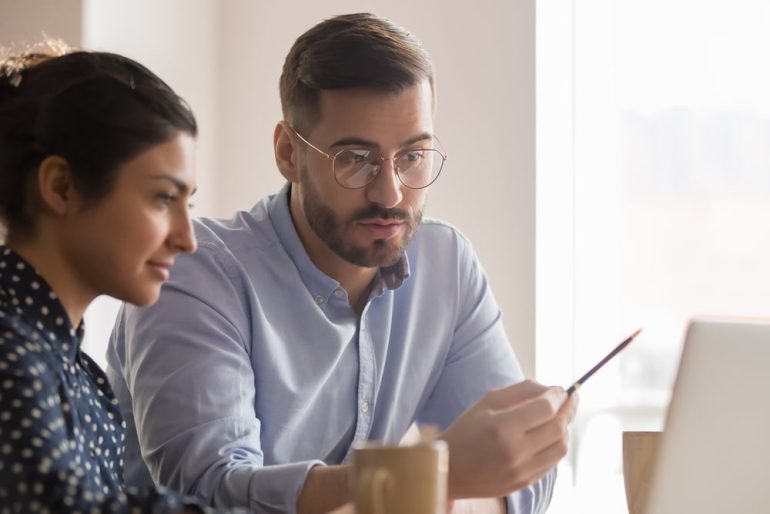
Training linguists on the fundamentals of post-edited machine translation will be crucial to your wider machine translation strategy.
Expectations will need to be aligned and managed, both for your linguists and your internal teams. A common misconception from linguists is that machine translation is supposed to replace human linguists when in fact, success is simply getting to a point where machine translation is actually good enough to be edited to a higher quality at a faster rate than translating the source text from scratch.
You’ll have a better chance of converting a translator to a post-editor by highlighting the expectation should be that there will be errors in the machine translation output and these errors will need correcting and improving by a linguist. And both linguists and internal teams should note that one of the important metrics is the productivity of the linguists doing the work.
Neural machine translation metrics
When it comes to machine translation, there are some key metrics to assess productivity and quality. In the development arena, metrics such as BLEU, TER and various others have been used to automatically attempt to assess the machine translation engine’s quality.
While these may be useful references, they are largely used by computer scientists. It’s at this point that you’ll want to have your linguists evaluate the machine translations to get a real flavour of the elegance of the output quality according to your quality criteria.
At TOPPAN Digital Language, we found it helpful to use two metrics when assessing machine translation viability in terms of quality and production; the post-edited distance (the distance between the final version of the translation after human editing versus the original machine-translation output) – and words per hour of the post-editor. The metrics that you will use depend on your context and what you’re looking to achieve.
While what we’ve detailed in this article is how you start your machine translation journey, you’ll have to keep in mind how it will scale. The key to success isn’t just about getting started, it’s also about how you learn to adapt!
Interested in adaptive neural machine translation? We offer machine translation consultancy that starts with an initial consultation, an analysis of your context and a feasibility assessment in which we can propose possible routes to implementation.
Contact us to find out more.